What’s the difference between RPA and Hyper-automation?
RPA focuses on automating specific tasks or processes using software robots, while hyper-automation aims to automate and optimize end-to-end processes using a combination of technologies, including RPA, AI, ML, and more.
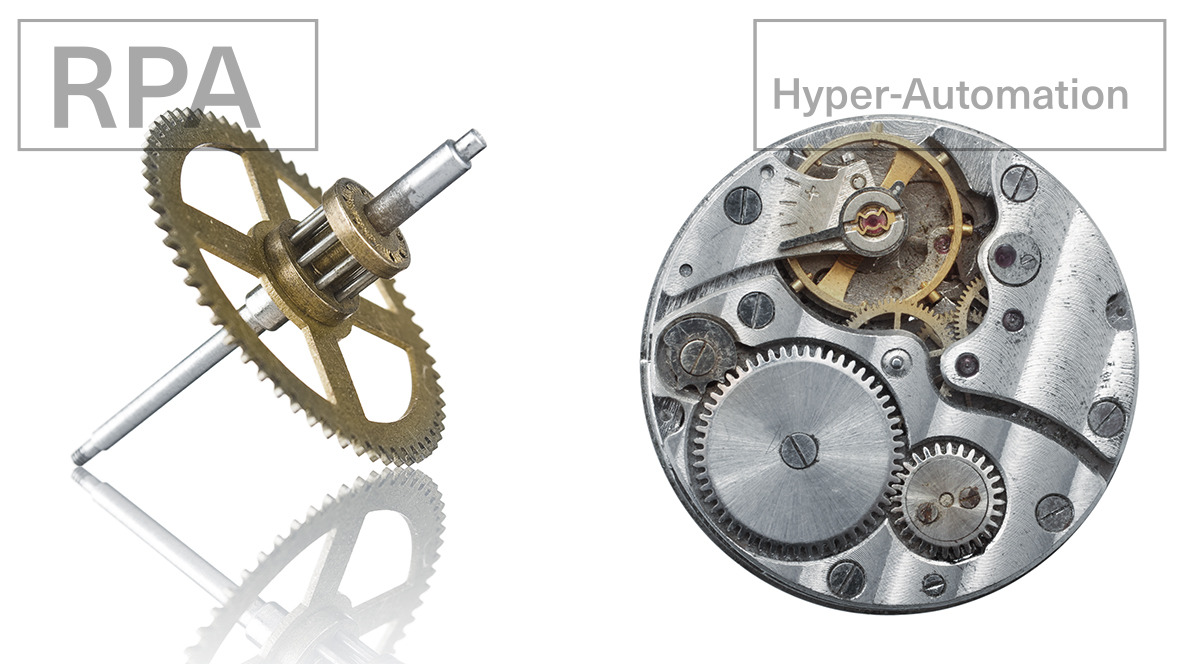
While RPA is a component of hyper-automation, hyper-automation encompasses a wider range of technologies and strategies to achieve more transformative and strategic automation outcomes. It’s about combining various automation tools to create intelligent and adaptable systems that can handle more complex tasks, adapt to changing conditions, and provide advanced analytics and insights for process improvement.
- Robotic Process Automation (RPA): RPA involves the use of software robots or “bots” to automate repetitive, rule-based tasks within existing business processes. These bots mimic human interactions with user interfaces, applications, and systems to perform tasks such as data entry, data extraction, form filling, and other similar activities. RPA focuses on automating specific tasks or processes to increase efficiency, reduce errors, and free up human workers from mundane work.
RPA is typically used for structured data and follows predefined rules and workflows. It does not require extensive programming or system integration since it operates at the user interface level. RPA tools can be trained or programmed to perform tasks, and they often rely on predefined rules or instructions to execute tasks accurately.
- Hyper-automation: Hyper-automation, on the other hand, is a broader and more advanced concept that goes beyond RPA. It combines several technologies, including RPA, artificial intelligence (AI), machine learning (ML), process mining, natural language processing (NLP), and more, to automate and optimize end-to-end business processes.
Hyper-automation aims to automate and optimize complex business processes that involve unstructured data, decision-making, and interactions across various systems and applications. It leverages AI and ML to enable cognitive capabilities, such as understanding and processing natural language, making predictions, and learning from data. It can automate both front-end and back-end processes and handle more sophisticated tasks that involve judgment, analysis, and adaptation.
Hyper-automation focuses on achieving holistic automation, connecting different systems and applications, orchestrating workflows, and enabling end-to-end process automation. It aims to achieve improved productivity, agility, accuracy, and scalability across the organization